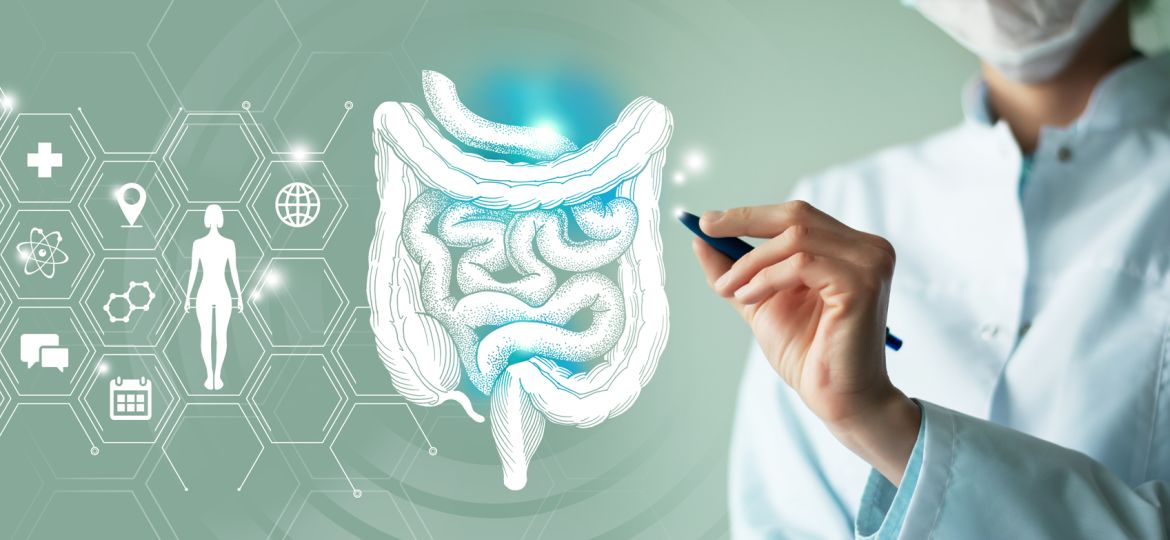
Despite minor differences, most national colorectal cancer (CRC) screening programs in Europe consist of one-size-fits-all aged-based strategies. These, however, have a low participation rate, with only 14% of susceptible EU citizens participating in CRC screening programs. As such, there is a clear need for both the accurate, non-invasive, cost-effective screening tests being developed by ONCOSCREEN, and for raising awareness about the disease and the importance of its early detection. One strategy may be by introducing incentive mechanisms to promote screening adoption.
It is clear that personalized screening strategies, that consider how behavioral, socioeconomic, and genetic factors influence the development of CRC, are needed. These then need to be paired with decision-support tools with guarantees that facilitate experts in their implementation in relevant contexts. Within the ONCOSCREEN project, the Mathematical Sciences Institute (ICMAT) of the Spanish National Research Council has proposed a two-step approach to developing a personalized strategy for each patient through modelling CRC risk.
First, a Bayesian Network model has been built using a large CRC-related dataset coming from an observational study that covers the annual health assessments of adult workers affiliated with a private health insurance provider, together with extensive medical experts’ guidance. This model draws on potential CRC risk factors (both modifiable and non-modifiable) and relevant medical conditions to assess the probability of having the disease. The model is used to rank these risk factors and pair them with an appropriate novel approach, as well as create risk maps that relate to these risk factors. Aside from age, which is perhaps the most predictive factor for CRC, the model points to risk factors such as diabetes, hypertension, and smoking and alcohol consumption. The risk maps generated characterize the differing effects that risk factors have on different population segments. The model can also be used to stratify population segments in terms of CRC risk. Full details may be seen in [1].
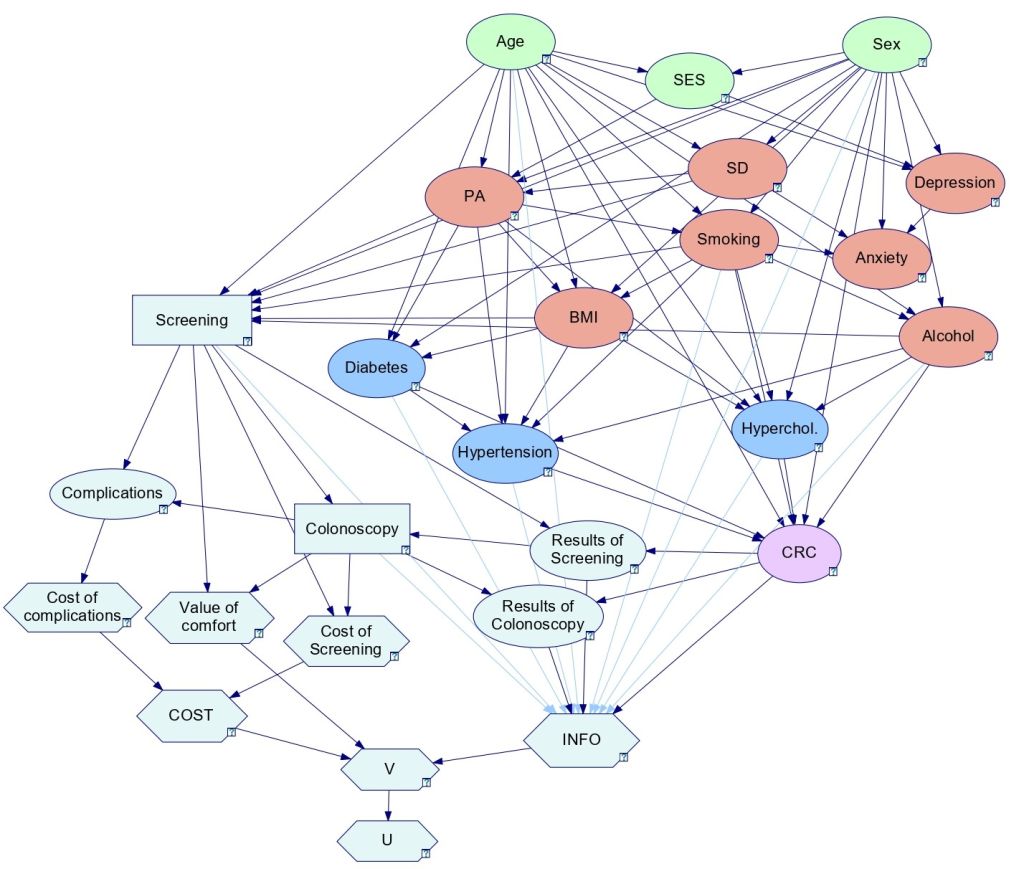
In a joint effort with the UNIRIOJA team, the CRC-risk model, developed by the Bayesian Network model, is complemented with an influence diagram model which identifies the possible screening strategies to be adopted, their possible impacts, and how these are to be assessed. The screening strategy that is suggested takes into account the implementation costs (including a colonoscopy, if necessary); the associated comfort level; the possible complications that may arise during the process; and the amount of information that each method can provide. A generic “parametrizable utility function” then aggregates the above criteria, taking into account how risk averse the corresponding decision maker is. Finally, a screening protocol is developed for an individual, as determined through a “maximum expected utility” algorithm. In doing so, several use cases are considered, including various examples underscoring the need for personalized screening strategies; the assessment of a national strategy showcasing how its results may be improved; the design of a national personalized screening policy; and, a methodology to assess and benchmark new screening tools and methods, such as those soon to be delivered in ONCOSCREEN. Full details may be seen in [2].
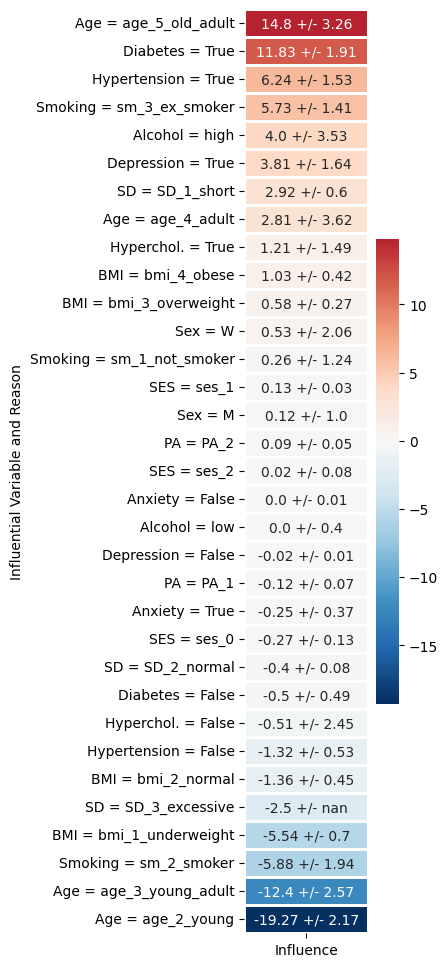
Software for both models is freely available for academic purposes from our github site.
As next steps, the decision support model will be enhanced to explore the impact of introducing incentives to increase acceptance and adherence within EU screening programs, incorporating principal-agent and adversarial risk analysis methods-. The aim is to achieve higher participation in CRC screening to facilitate earlier detection. We are also anxiously looking forward to benchmarking the new ONCOSCREEN devices through the above models, once they are ready. Stay tuned!!
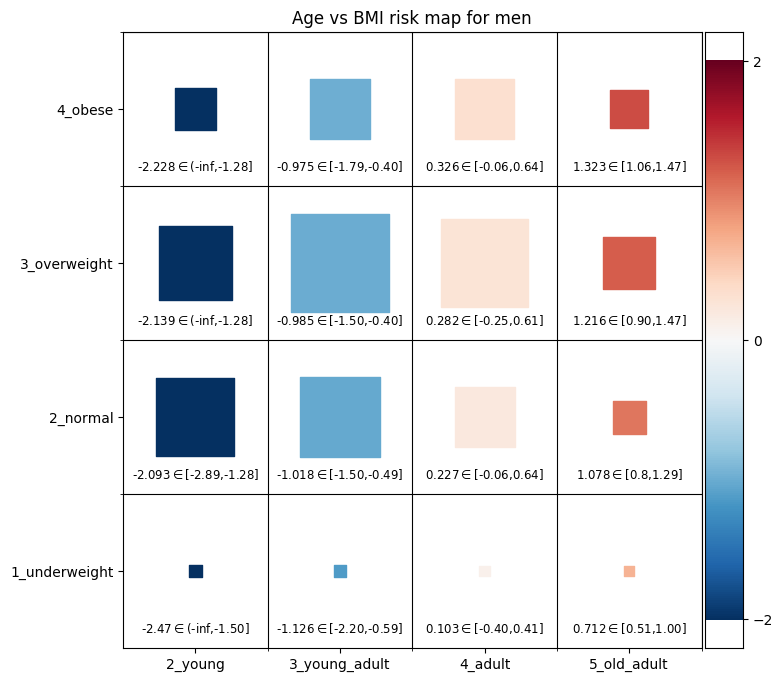
References
[1] Corrales, D., Santos-Lozano, A., López-Ortiz, S., Lucia, A., & Insua, D. R. (2024). Colorectal cancer risk mapping through Bayesian networks. Computer Methods and Programs in Biomedicine, 257, 108407.
[2] Corrales, D., Insua, D. R., & González, M. J. (2025). A decision analysis model for colorectal cancer screening. arXiv preprint arXiv:2502.21210.
[3] https://www.sciencedirect.com/science/article/abs/pii/S0016508523001609
Written by Daniel Corrales and David Rios Insua (ICMAT-CSIC, Madrid, Spain)