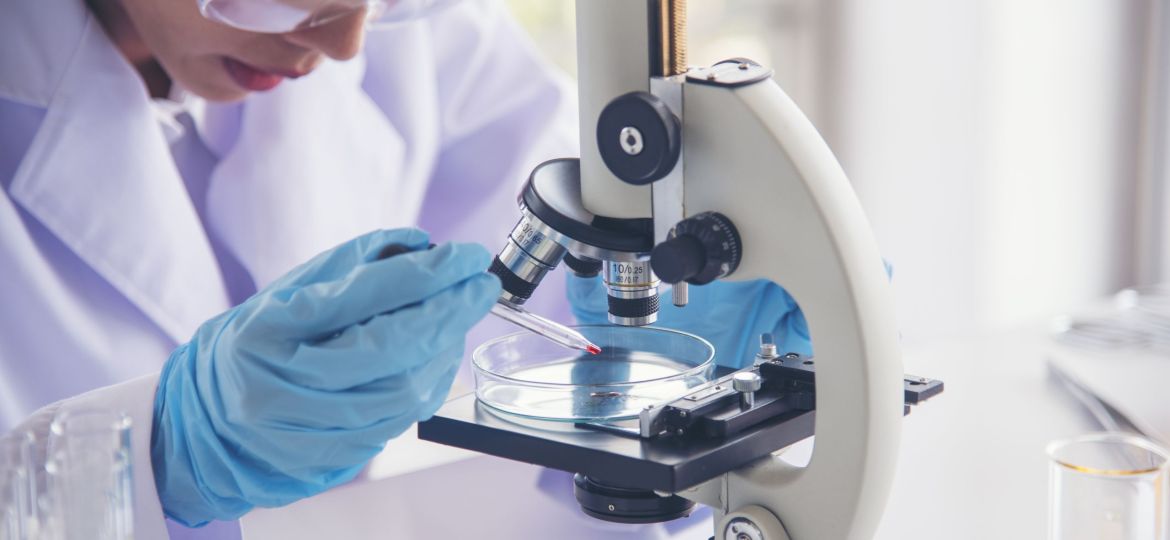
Colorectal cancer (CRC) is one of the most common and lethal forms of cancer worldwide. Its early detection and effective treatment are critical for improving patient outcomes and its detection depends heavily on the medical experts, more precisely pathologists, who examine the suspicious tissue samples for CRC. While traditional diagnostic and therapeutic methods still face limitations in accuracy, efficiency, and accessibility, the transformation in pathology to digital pathology and algorithms can now be used to analyse medical videos and images, which are called Whole Slide Images (WSI).
Artificial intelligence (AI) has emerged as a transformative tool in the fight against CRC. By leveraging advanced machine learning algorithms, AI systems can already analyse medical images to support pathologists with additional information during the diagnostic process. Possible AI applications in the assessment of WSIs from CRC suspected cases include the detection of polyps, glands and tissue types, the prediction of patient outcomes, and the classification into different stages of the disease.
These AI algorithms are trained using medical data, including annotated images provided by pathologists. Pathologists play a critical role in this process by labelling the medical images, to highlight regions of interest, such as tumours, polyps, or other abnormalities, which enables the training of the AI models.
But how does the expert assess these medical images to make annotations? The current focus in research is on learning from the annotations, but not the process by which these annotations and medical decisions are defined. This shift in focus is significant as these findings could not only be used to improve the decision making of existing AI algorithms, but, above all, to support and accelerate the learning process from trainee to expert within pathology. Within the ONCOSCREEN project, the Medical University of Graz in Austria, are asking the question: how do pathologists examine WSIs and how do they navigate through these very large images while making a diagnosis?
To get to the bottom of this question, a team, led by Dr. Heimo Müller, use the causability lab, which contains an eye tracking setup that captures the gaze points of participants working on a computer screen (i.e. what part of the image the participant is looking at). These participants consist of pathologists with varying degrees of expertise in the assessment of CRC WSIs. They are therefore both young doctors with less experience in this specific field, as well as experts with years of practical experience in the diagnosis of suspected CRC cases.
In addition to this, ONCOSCREEN is also looking at the navigation behaviour of pathologists to be able to draw conclusions about the regions of interest that the identify with the WSIs, as this defines how these medical experts formulate a correct diagnosis. We described the region of interest as the “essential image sections”, which are what a pathologist looks at in order to formulate their diagnosis based on the WSI. This can be not only the relevant regions with potential cancer, but also neighbouring tissue areas or for an overview of the overall appearance of the WSI. The information that will be obtained should enable an understanding of the key regions in a WSI based on the observation behaviour of human experts. The goal is to provide young aspiring doctors with these findings to help them advance from trainee to expert.
In the next step, ONCOSCREEN will begin to train the algorithm that recognizes patterns in the pathologists’ viewpoints based on that data. These findings will form the basis of the learning tool, in which experts essentially pass on their knowledge and trainers and trainees can expand their experience, knowledge for the observation of WSIs, and the formulation of diagnoses. In the future, this will enable us to gain deeper insights and knowledge about the diagnostic process from experienced pathologists and support as well as improve the early detection of CRC.